Williams, Eric
(2013)
Automated Detection of Anomalous Patterns in Validation Scores for Protein X-Ray Structure Models.
Doctoral Dissertation, University of Pittsburgh.
(Unpublished)
This is the latest version of this item.
![[img]](http://d-scholarship.pitt.edu/style/images/fileicons/text_plain.png) |
Plain Text (A1: wine quality rules, 3 bins)
Supplemental Material
Download (9kB)
|
![[img]](http://d-scholarship.pitt.edu/style/images/fileicons/text_plain.png) |
Plain Text (A2: wine quality rules, 10 bins)
Supplemental Material
Download (11kB)
|
![[img]](http://d-scholarship.pitt.edu/style/images/fileicons/text_plain.png) |
Plain Text (B1: SECOM rules, 3 bins)
Supplemental Material
Download (2kB)
|
![[img]](http://d-scholarship.pitt.edu/style/images/fileicons/text_plain.png) |
Plain Text (B2: SECOM rules, 10 bins)
Supplemental Material
Download (4kB)
|
![[img]](http://d-scholarship.pitt.edu/style/images/fileicons/text_plain.png) |
Plain Text (C1: total crime rules, 3 bins)
Supplemental Material
Download (3kB)
|
![[img]](http://d-scholarship.pitt.edu/style/images/fileicons/text_plain.png) |
Plain Text (C2: total crime rules, 10 bins)
Supplemental Material
Download (5kB)
|
![[img]](http://d-scholarship.pitt.edu/style/images/fileicons/text_plain.png) |
Plain Text (D1: cardiotocography rules, 3 bins)
Supplemental Material
Download (5kB)
|
![[img]](http://d-scholarship.pitt.edu/style/images/fileicons/text_plain.png) |
Plain Text (D2: cardiotocography rules, 10 bins)
Supplemental Material
Download (4kB)
|
![[img]](http://d-scholarship.pitt.edu/19601/9.hassmallThumbnailVersion/SuppE_complete_PDB_attribute_list.pdf) 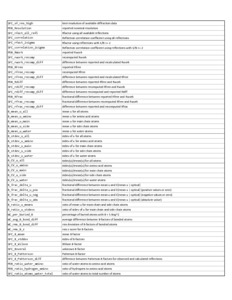 Preview |
|
PDF (E: complete PDB attribute list)
Supplemental Material
Download (42kB)
| Preview
|
![[img]](http://d-scholarship.pitt.edu/style/images/fileicons/text_plain.png) |
Plain Text (F: missing PDB values summaries)
Supplemental Material
Download (23kB)
|
![[img]](http://d-scholarship.pitt.edu/style/images/fileicons/text_plain.png) |
Plain Text (G: PDB attribute semantic groupings)
Supplemental Material
Download (3kB)
|
![[img]](http://d-scholarship.pitt.edu/style/images/fileicons/text_plain.png) |
Plain Text (H: deep PDB inlier ids)
Supplemental Material
Download (1kB)
|
![[img]](http://d-scholarship.pitt.edu/style/images/fileicons/text_plain.png) |
Plain Text (I: extreme PDB outlier ids)
Supplemental Material
Download (1kB)
|
![[img]](http://d-scholarship.pitt.edu/19601/14.hassmallThumbnailVersion/SuppJ_validation_score_values_for_maximal_outliers.pdf) 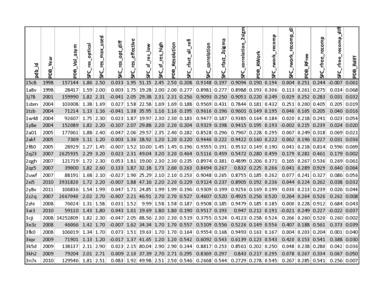 Preview |
|
PDF (J: validation scores for maximal outliers)
Supplemental Material
Download (136kB)
| Preview
|
![[img]](http://d-scholarship.pitt.edu/style/images/fileicons/text_plain.png) |
Plain Text (K: select PDB attribute value summaries)
Supplemental Material
Download (7kB)
|
![[img]](http://d-scholarship.pitt.edu/style/images/fileicons/text_plain.png) |
Plain Text (L: PDB rules, w/o dummy class)
Supplemental Material
Download (56kB)
|
![[img]](http://d-scholarship.pitt.edu/style/images/fileicons/text_plain.png) |
Plain Text (M: PDB rules, w/ dummy class)
Supplemental Material
Download (22kB)
|
![[img]](http://d-scholarship.pitt.edu/style/images/fileicons/text_plain.png) |
Plain Text (N: extreme PDB outlier rules)
Supplemental Material
Download (34kB)
|
![[img]](http://d-scholarship.pitt.edu/style/images/fileicons/text_plain.png) |
Plain Text (O: deep PDB inlier rules)
Supplemental Material
Download (32kB)
|
![[img]](http://d-scholarship.pitt.edu/19601/61.hassmallThumbnailVersion/Eric_Williams_dissertation_%28revised%29.pdf) 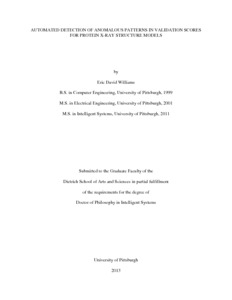 Preview |
|
PDF (dissertation)
Primary Text
Download (1MB)
| Preview
|
Abstract
Structural bioinformatics is a subdomain of data mining focused on identifying structural patterns relevant to functional attributes in repositories of biological macromolecular structure models. This research focused on structures determined via x-ray crystallography and deposited in the Protein Data Bank (PDB).
Protein structures deposited in the PDB are products of experimental processes, and only approximately model physical reality. Structural biologists address accuracy and precision concerns via community-enforced consensus standards of accepted practice for proper building, refinement, and validation of models. Validation scores are quantitative partial indicators of the likelihood that a model contains serious systematic errors.
The PDB recently convened a panel of experts, which placed renewed emphasis on troubling anomalies among deposited structure models. This study set out to detect such anomalies. I hypothesized that community consensus standards would be evident in patterns of validation scores, and deviations from those standards would appear as unusual combinations of validation scores.
Validation attributes were extracted from PDB entry headers and multiple software tools (e.g., WhatCheck, SFCheck, and MolProbity). Independent component analysis (ICA) was used for attribute transformation to increase contrast between inliers and outliers. Unusual patterns were sought in regions of locally low density in the space of validation score profiles, using a novel standardization of Local Outlier Factor (LOF) scores.
Validation score profiles associated with the most extreme outlier scores were demonstrably anomalous according to domain theory. Among these were documented fabrications, possible annotation errors, and complications in the underlying experimental data. Analysis of deep inliers revealed promising support for the hypothesized link between consensus standard practices and common validation score values.
Unfortunately, with numerical anomaly detection methods that operate simultaneously on numerous continuous-valued attributes, it is often quite difficult to know why a case gets a particular outlier score. Therefore, I hypothesized that IF-THEN rules could be used to post-process outlier scores to make them comprehensible and explainable. Inductive rule extraction was performed using RIPPER. Results were mixed, but they represent a promising proof of concept.
The methods explored are general and applicable beyond this problem. Indeed, they could be used to detect structural anomalies using physical attributes.
Share
Citation/Export: |
|
Social Networking: |
|
Details
Item Type: |
University of Pittsburgh ETD
|
Status: |
Unpublished |
Creators/Authors: |
|
ETD Committee: |
|
Date: |
18 October 2013 |
Date Type: |
Publication |
Defense Date: |
21 June 2013 |
Approval Date: |
18 October 2013 |
Submission Date: |
1 August 2013 |
Access Restriction: |
1 year -- Restrict access to University of Pittsburgh for a period of 1 year. |
Number of Pages: |
226 |
Institution: |
University of Pittsburgh |
Schools and Programs: |
Dietrich School of Arts and Sciences > Intelligent Systems |
Degree: |
PhD - Doctor of Philosophy |
Thesis Type: |
Doctoral Dissertation |
Refereed: |
Yes |
Uncontrolled Keywords: |
outlier detection, anomaly detection, protein, x-ray crystallography, structure, model, validation, rule extraction |
Date Deposited: |
18 Oct 2013 16:32 |
Last Modified: |
15 Nov 2016 14:14 |
URI: |
http://d-scholarship.pitt.edu/id/eprint/19601 |
Available Versions of this Item
-
Automated Detection of Anomalous Patterns in Validation Scores for Protein X-Ray Structure Models. (deposited 18 Oct 2013 16:32)
[Currently Displayed]
Metrics
Monthly Views for the past 3 years
Plum Analytics
Actions (login required)
 |
View Item |