Thomas, Christopher
(2020)
Modeling Visual Rhetoric and Semantics in Multimedia.
Doctoral Dissertation, University of Pittsburgh.
(Unpublished)
![[img]](http://d-scholarship.pitt.edu/39501/25.hassmallThumbnailVersion/Chris_Thomas_Dissertation.pdf) 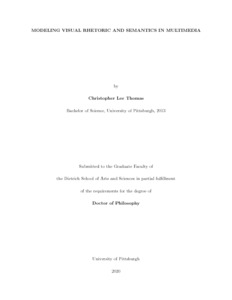 Preview |
|
PDF (Christopher Lee Thomas Dissertation - Modeling Visual Rhetoric and Semantics in Multimedia)
Primary Text
Download (12MB)
| Preview
|
Abstract
Recent advances in machine learning have enabled computer vision algorithms to model complicated visual phenomena with accuracies unthinkable a mere decade ago. Their high-performance on a plethora of vision-related tasks has enabled computer vision researchers to begin to move beyond traditional visual recognition problems to tasks requiring higher-level image understanding. However, most computer vision research still focuses on describing what images, text, or other media literally portrays. In contrast, in this dissertation we focus on learning how and why such content is portrayed. Rather than viewing media for its content, we recast the problem as understanding visual communication and visual rhetoric. For example, the same content may be portrayed in different ways in order to present the story the author wishes to convey. We thus seek to model not only the content of the media, but its authorial intent and latent messaging. Understanding how and why visual content is portrayed a certain way requires understanding higher level abstract semantic concepts which are themselves latent within visual media. By latent, we mean the concept is not readily visually accessible within a single image (e.g. right vs left political bias), in contrast to explicit visual semantic concepts such as objects.
Specifically, we study the problems of modeling photographic style (how professional photographers portray their subjects), understanding visual persuasion in image advertisements, modeling political bias in multimedia (image and text) news articles, and learning cross-modal semantic representations. While most past research in vision and natural language processing studies the case where visual content and paired text are highly aligned (as in the case of image captions), we target the case where each modality conveys complementary information to tell a larger story. We particularly focus on the problem of learning cross-modal representations from multimedia exhibiting weak alignment between the image and text modalities. A variety of techniques are presented which improve modeling of multimedia rhetoric in real-world data and enable more robust artificially intelligent systems.
Share
Citation/Export: |
|
Social Networking: |
|
Details
Item Type: |
University of Pittsburgh ETD
|
Status: |
Unpublished |
Creators/Authors: |
|
ETD Committee: |
|
Date: |
16 September 2020 |
Date Type: |
Publication |
Defense Date: |
14 July 2020 |
Approval Date: |
16 September 2020 |
Submission Date: |
2 August 2020 |
Access Restriction: |
No restriction; Release the ETD for access worldwide immediately. |
Number of Pages: |
220 |
Institution: |
University of Pittsburgh |
Schools and Programs: |
Dietrich School of Arts and Sciences > Computer Science |
Degree: |
PhD - Doctor of Philosophy |
Thesis Type: |
Doctoral Dissertation |
Refereed: |
Yes |
Uncontrolled Keywords: |
computer vision, visual rhetoric, semantics, visual recognition, image understanding, retrieval, classification, cross-modal, vision, language |
Date Deposited: |
16 Sep 2020 15:09 |
Last Modified: |
16 Sep 2020 15:09 |
URI: |
http://d-scholarship.pitt.edu/id/eprint/39501 |
Metrics
Monthly Views for the past 3 years
Plum Analytics
Actions (login required)
 |
View Item |